With movies like ‘Terminator’, ‘I, Robot’, ‘Ex Machina’ and ‘The Matrix’ dominating Artificial Intelligence (AI) pop culture, the average consumer’s initial prerogative is to assume that AI is a threat to the world’s security. Who can blame them? In these films, we witness the (hopefully!) hyperbolized impact AI can have when its capabilities and growth are left unchecked – and it’s not pretty. In reality, current AI technology is far from the level we see in something like ‘The Matrix’ – a self-sustained entity that possesses Artificial General Intelligence (AGI). However, it’s still important to look at how Cybersecurity and AI can interoperate both now and in the future.
First, let’s take a look at how cybersecurity can become more fragile with the increasing public accessibility of powerful AI models. There have been valid concerns that these models in the hands of bad actors will give them the ability to defeat existing security technologies. Over the last twelve months, 85% of security experts that have noted an increase in cyber attacks attributed the surge to generative AI.
For starters, Large Language Models (LLMs) such as OpenAI’s ChatGPT and Google’s Bard greatly reduce the barrier to entry for non-technical individuals with an intention to commit cyber crimes. Being able to learn high-level knowledge about network security protocols, previous vulnerabilities and automation provides anyone with enough malicious intent the tools necessary to become a threat. Beyond that, LLMs make it easier for attackers to leverage generative AI to increase the scale of their crimes; generating phishing scams, writing malware, and creating social engineering attack dialogue can be done in a fraction of the time it used to take. Hackers from foreign countries were often held back by their lack of proficiency in using the English language, which led to their phishing attacks sounding just that – fishy. Now, however, people with bad written skills can come up with dialogue and content that sounds intelligible to the untrained eye.
Hackers often use social engineering to compromise an individual’s privacy and assets, and many businesses emphasize, in their employee cybersecurity guidelines, the importance of vetting any and all communications to ensure its authenticity. With the recent advancements in machine learning technology, individuals need to be even more careful when detecting social engineering attempts. For instance, it is now fairly cheap and easy to create an audio deepfake of another person’s voice which means your grandmother calling you asking for your bank password, could very well not be your grandmother! These audio deepfakes will only get more refined as time goes on, so it is important to put measures in place to fend off these kinds of attacks. Try setting a verbal password between yourself and those close to you!
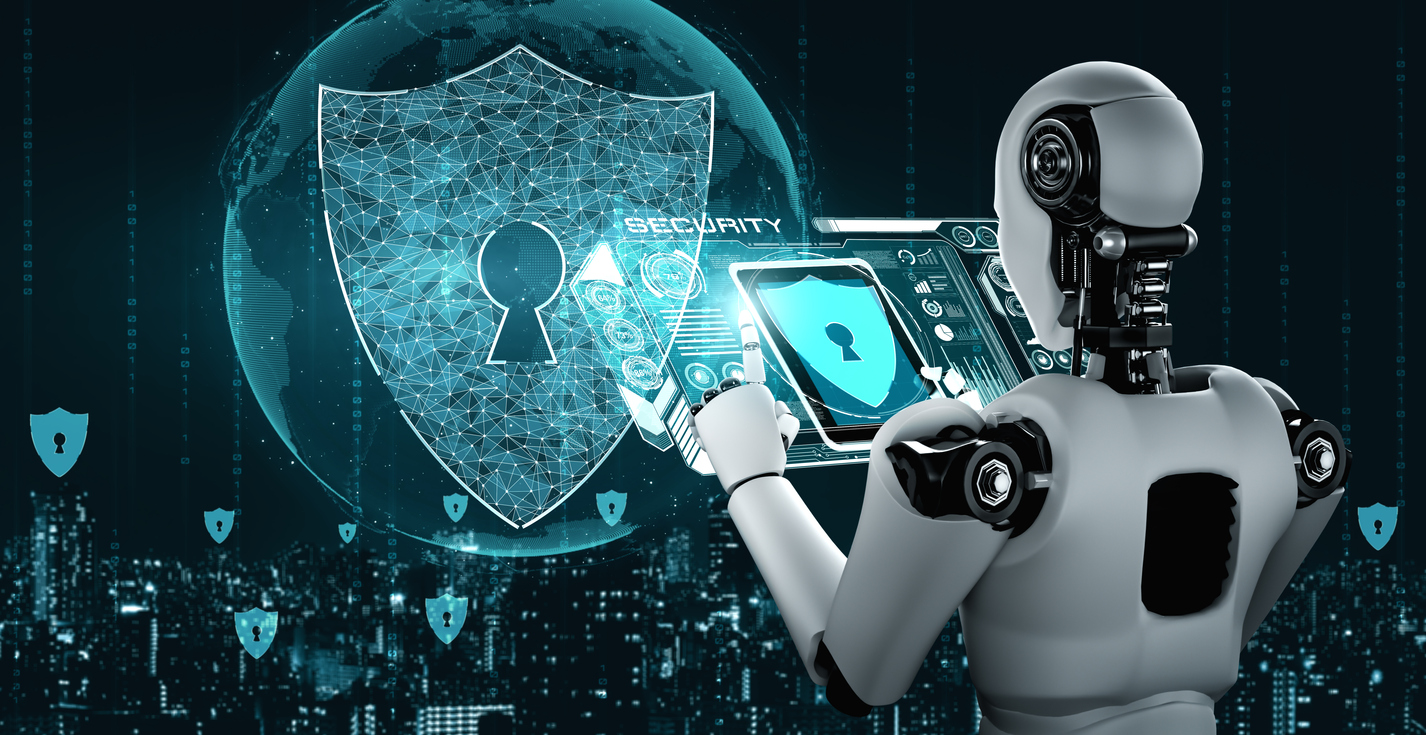
The threat posed by AI isn’t limited to just the vectors by which AI can boost an attacker’s capabilities. The training of models requires vast amounts of data in order to fine tune the technology into a state that boasts high accuracy. Depending on the function of the model, the data used for training can be highly sensitive. This leads us to several questions: Where does AI data reside? Who can access it? What happens when the data is no longer needed? Data privacy will need to continue to become a focus for organizations that hope to leverage AI in their products and services.
Now, before you decide to condemn AI and any further advances in the field, it’s important to remember that AI can also have a positive impact on security. Machine learning, predictive analytics and natural language processing can be strong tools when used in the right ways – so much so that 82% of IT decision-makers plan to invest in AI-driven cybersecurity in the next two years.
When used correctly, AI can be powerful in preventing attacks before they occur. Predictive analysis in conjunction with natural language processing allows security specialists to scrape the web at an extremely large scale to keep up to date on emerging cyber threats. This, in turn, allows one to be more prepared for novel vulnerabilities that have not yet become prominent in the industry. Furthermore, while some humans can outperform machine learning models at cognitive analysis, the ability of AI algorithms to ingest, organize and analyze massive volumes of data make them much more adept at pattern recognition. This can be useful in the early detection of anomalistic behavior, allowing IT engineers to prevent attacks before they even enter their system.
But what if the system’s been compromised already? Not to worry, generative AI can also be used in incidence response. Instead of spending a copious amount of human hours analyzing vast amounts of data and reverse engineering malware infections, AI models can automatically scan code, network traffic, and disk-level corruption and provide insights that are useful when trying to understand the implications and behavior of a cyber attack. This leaves more time for human engineers to provide their skills in areas that cannot be handled by AI. There was nearly $6,000,000,000 of private AI-focused financing that went into the cybersecurity industry in the last year – so the value of using AI should not be understated.
So what now? Do we pull the plug on AI, or do we go full speed ahead? Like with all complex technologies, the answer lies in finding the right balance. The AI genie can’t be put back in the bottle, so it is up to us to ensure we’re putting the right precautions in place to ensure its capabilities don’t grow beyond our control, while simultaneously not preventing ourselves from reaping the benefits of such a powerful technology.
References: Security Magazine, Electronic Frontier Foundation, Blackberry, Statista